A paper published by LyRIDS professors is selected to figure as the cover issue on Journal of Sensor and Actuator Networks (JSAN)
The paper intitled “A Multi-Agent Intrusion Detection System Optimized by a Deep Reinforcement Learning Approach with a Dataset Enlarged Using a Generative Model to Reduce the Bias Effect” was recently published on JSAN Journal. The authors of this paper are Dr. Jun Kim and Dr. Medeiros Machado both Associate Professors at the Lyrids Laboratory. This paper was written based on the results obtained by the student Matthieu Mouyart during his internship in the Lyrids lab. Due to the quality of the paper the journals’ editor has chosen it to figure as the cover issue of the volume 12 issue 5.
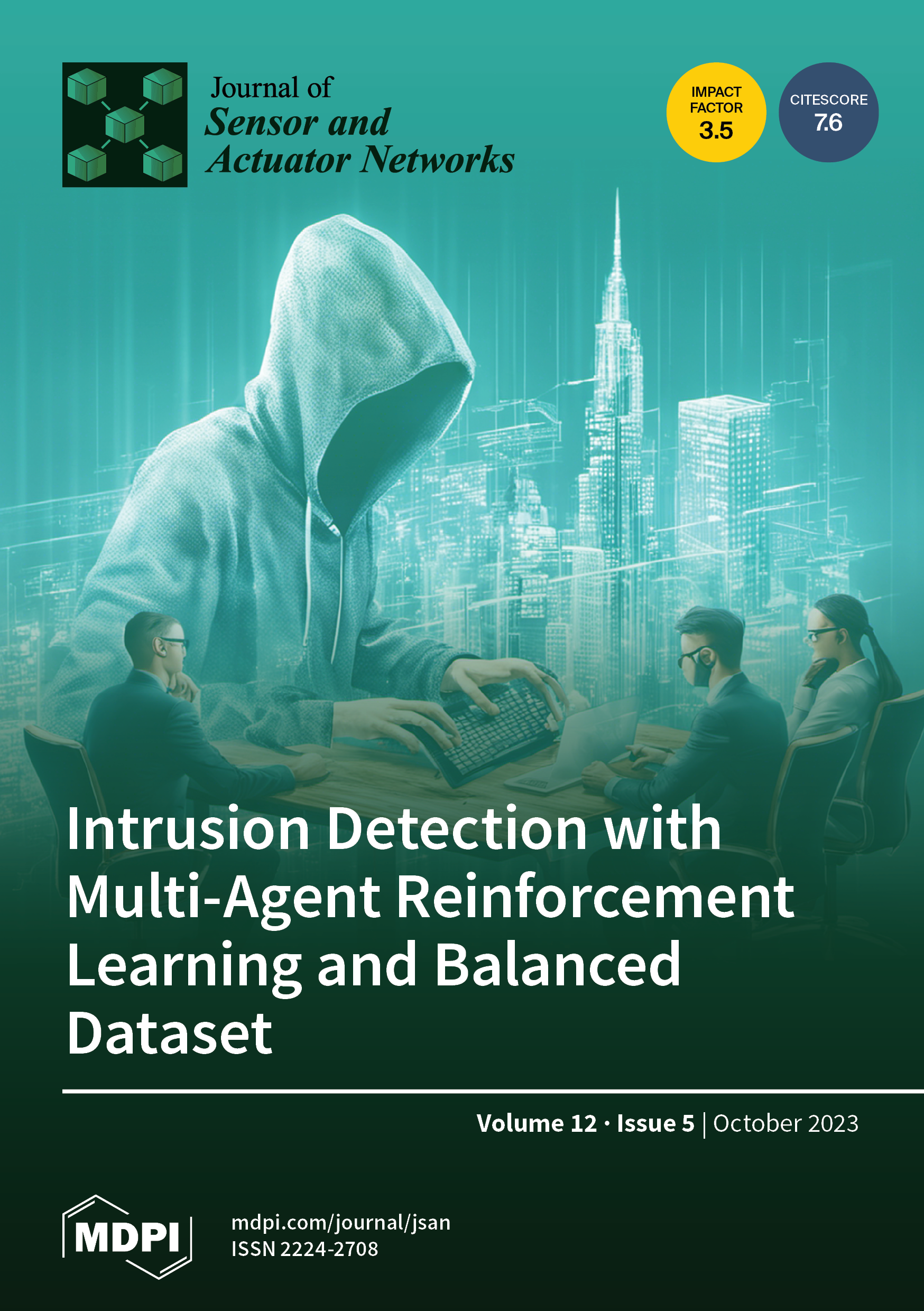
The paper subject is described below:
Intrusion detection systems are often calibrated with datasets that are unbalanced in terms of attack data and non-attack data causing bias effects, and therefore making them vulnerable to cyberattacks. As an approach to remedy this issue, we considered the Conditional Tabular Generative Adversarial Network (CTGAN) to balance an insider threat tabular dataset called the CMU-CERT. We generated insider threats using the CTGAN and combined them with the original data. We used the resulting dataset for an intrusion detection system implemented with Adversarial Environment Reinforcement Learning (AE-RL) in a multi-agent framework with an attacker and a defender. We showed that the performance of detecting intrusions using the AE-RL is significantly improved when the dataset is balanced.